AI Trust, Risk, and Security Management (AI TRiSM)
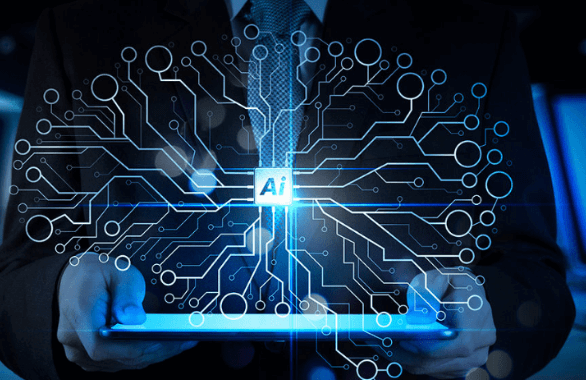
1. Introduction to AI TRiSM
Artificial Intelligence (AI) has rapidly integrated into various aspects of human life, revolutionizing industries like healthcare, finance, transportation, and communication. However, as AI systems become more pervasive, ensuring their trustworthiness, managing associated risks, and securing them against threats is essential. This convergence of efforts in trust, risk, and security management for AI systems is what the concept of AI Trust, Risk, and Security Management (AI TRiSM) represents.
AI TRiSM is a comprehensive framework designed to address the challenges organizations face when deploying AI technologies. It emphasizes building trust in AI systems, mitigating risks that may arise from their use, and securing them against potential cyberattacks or misuse. As AI systems evolve, so do the complexities surrounding their management, making AI TRiSM an indispensable tool for any modern enterprise.
2. Key Components of AI TRiSM
AI TRiSM is built on three fundamental pillars: Trust, Risk, and Security. These components form the foundation of how organizations should handle AI systems from development to deployment.
- Trust: Refers to the confidence stakeholders place in the AI system’s behavior, ethics, and decision-making processes.
- Risk: Involves identifying, assessing, and managing the risks that come with the use of AI, including operational, ethical, and reputational risks.
- Security: Focuses on protecting AI systems from cyber threats, ensuring the integrity, confidentiality, and availability of AI-driven processes.
Together, these pillars help organizations ensure that AI technologies are used responsibly, reliably, and securely.
See also: apkipl
3. AI Trust: Building Confidence in AI Systems
Trust in AI systems is paramount for widespread adoption and successful integration into business operations. Without trust, even the most sophisticated AI systems may be rejected by users, customers, and regulators. Several factors influence AI trust:
- Ethical AI: AI must adhere to ethical standards, ensuring it respects human rights, avoids biases, and operates fairly.
- Transparency and Explainability: Users must understand how AI makes decisions. This means that AI systems should be explainable, so that end-users, stakeholders, and regulators can trust its outputs.
- AI Fairness: Algorithms should ensure impartial decision-making, free from discrimination against any group based on race, gender, or socioeconomic status.
Building AI trust involves continuous communication, transparency, and ethical considerations. Organizations must demonstrate that AI systems act in ways that align with societal values and legal standards.
4. AI Risk: Identifying and Managing AI Risks
AI systems, while highly beneficial, are not without their risks. Risks in AI can be categorized broadly into three types:
- Operational Risks: This includes the risk of AI systems failing to perform as intended due to bugs, data issues, or model degradation over time.
- Ethical Risks: Concerns over AI systems making decisions that could harm individuals or propagate biases. Ethical issues also arise when AI systems invade privacy or make decisions without human oversight.
- Security Risks: These refer to threats like data breaches, model hacking, or adversarial attacks that compromise the integrity of AI systems.
To mitigate these risks, organizations must implement rigorous risk management strategies. This includes performing regular AI audits, setting up contingency plans, and ensuring that AI models are continuously monitored and updated based on performance.
5. AI Security: Safeguarding AI Systems
As AI systems grow more complex, so do the vulnerabilities associated with them. AI security addresses the methods used to protect these systems from cyber threats. AI systems are attractive targets for hackers due to their reliance on large datasets and critical decision-making capabilities. Several aspects of AI security include:
- AI System Vulnerabilities: From data manipulation to adversarial attacks, AI models can be easily tricked by malicious actors if not properly secured.
- Cybersecurity Measures for AI: Implementing encryption, access controls, and secure model training techniques can help safeguard AI systems.
- AI in Cyber Defense: AI is also being used in cybersecurity to detect, predict, and respond to cyber threats. Machine learning algorithms can quickly analyze vast amounts of data to identify anomalies and potential breaches in real-time.
Securing AI systems requires a combination of traditional cybersecurity measures and AI-specific protections, such as defenses against adversarial attacks.
6. Governance in AI TRiSM
Effective AI governance is critical for managing AI trust, risk, and security. Governance frameworks help ensure that AI systems are developed and deployed responsibly. Key aspects of AI governance include:
- AI Governance Frameworks: Establishing structures and processes to ensure AI systems adhere to ethical guidelines, legal standards, and business objectives.
- Roles of AI Governance Committees: Committees made up of diverse stakeholders (e.g., data scientists, ethicists, legal experts) should oversee AI development and deployment.
- Regulatory Compliance: Ensuring AI systems comply with laws like the General Data Protection Regulation (GDPR) or the California Consumer Privacy Act (CCPA).
Governance in AI TRiSM helps bridge the gap between technology and regulation, ensuring AI aligns with both business goals and societal values.
7. The Role of Ethics in AI TRiSM
Ethics is a core consideration in AI TRiSM. AI technologies must operate within the boundaries of accepted moral principles. Ethical AI can be defined by several factors:
- Ethical Guidelines for AI: Guidelines ensure AI operates in ways that are morally acceptable and do not harm users or society.
- Ensuring AI Fairness: AI systems should provide equitable outcomes and not discriminate against individuals or groups.
- Bias Mitigation Techniques: Methods like dataset balancing, fairness-aware algorithms, and post-hoc bias corrections can reduce bias in AI decision-making.
Ethical considerations in AI are not just a legal obligation but a moral one. Addressing them helps build trust and ensures that AI serves humanity in beneficial ways.
8. AI Bias and Discrimination
One of the biggest challenges in AI systems is the presence of bias. Bias in AI can occur at any stage, from data collection to algorithm design. There are several types of bias in AI systems, including:
- Data Bias: Occurs when training data is unrepresentative of the real-world population or contains historical biases.
- Algorithmic Bias: Arises when an AI model amplifies biases present in the data or misinterprets patterns.
- Discriminatory Outcomes: These are biased results that can affect hiring decisions, credit approvals, or law enforcement applications, leading to unfair treatment of certain groups.
To tackle bias, organizations must implement robust mechanisms for detecting, analyzing, and addressing it. Legal and regulatory frameworks are also evolving to ensure that biased AI systems face accountability.
9. AI Transparency and Explainability
Transparency and explainability are crucial in establishing trust in AI systems. An AI system must not only produce accurate results but also provide understandable reasoning for its decisions. Key considerations include:
- What is AI Explainability?: The ability of an AI system to explain how and why a decision was made.
- Why It Matters for Trust: Explainable AI helps users understand and trust decisions, especially in critical areas like healthcare or finance.
- Models and Methods for Transparent AI: Techniques such as LIME (Local Interpretable Model-agnostic Explanations) and SHAP (SHapley Additive exPlanations) offer insights into how AI models generate predictions.
Transparency and explainability are not just technical requirements but also crucial for regulatory compliance and user trust.
10. Managing Operational Risks in AI
Operational risks arise when AI systems fail to perform as expected. These risks can be caused by a variety of factors, such as:
- AI Model Failures: Models may fail due to poor data quality, incorrect assumptions, or environmental changes.
- Contingency Planning for AI: Organizations must develop fallback mechanisms in case AI systems malfunction, such as human-in-the-loop interventions.
- Continuous Monitoring and AI Audits: Regular audits of AI systems help identify potential failures before they become critical.
Managing operational risks requires constant vigilance and the establishment of processes that ensure AI systems remain reliable and effective over time.